Climate models
Climate models can be a useful tool for real-world decision-making. Before using them in this way, however, it is important to understand how scientists make the models, how they are meant to be used, and their strengths and weaknesses. This section will illuminate these topics, and empower you to use data produced by climate models in an informed and risk-aware manner.
Scientists created climate models to better understand how the climate would change in response to changes in the composition of the atmosphere. At their most basic level, these models are based on fundamental laws of physics, simulate proven physical dynamics that govern atmospheric behavior, and reproduce patterns in observed data. Climate models simulate properties and processes across the full Earth system, including land, glaciers, forests, oceans, and the atmosphere.
The climate is a set of conditions that hold over seasons and years, while weather is made up of short-term atmospheric outcomes that can change within a day. Climate is expressed in ranges and averages, while weather is a precise phenomenon at a specific time in a specific place. Climate models thus offer helpful information about the trajectory and magnitude of climate outcomes for given changes in the atmosphere, but they are not meant to make precise predictions.
Climate models are created and maintained by scientific institutions around the world. Since models bring together many different forces that produce our complex atmosphere, the models require teams of experts working together. Most commonly, this happens in national laboratories and large research institutes.
CMIP5 Modeling Centers
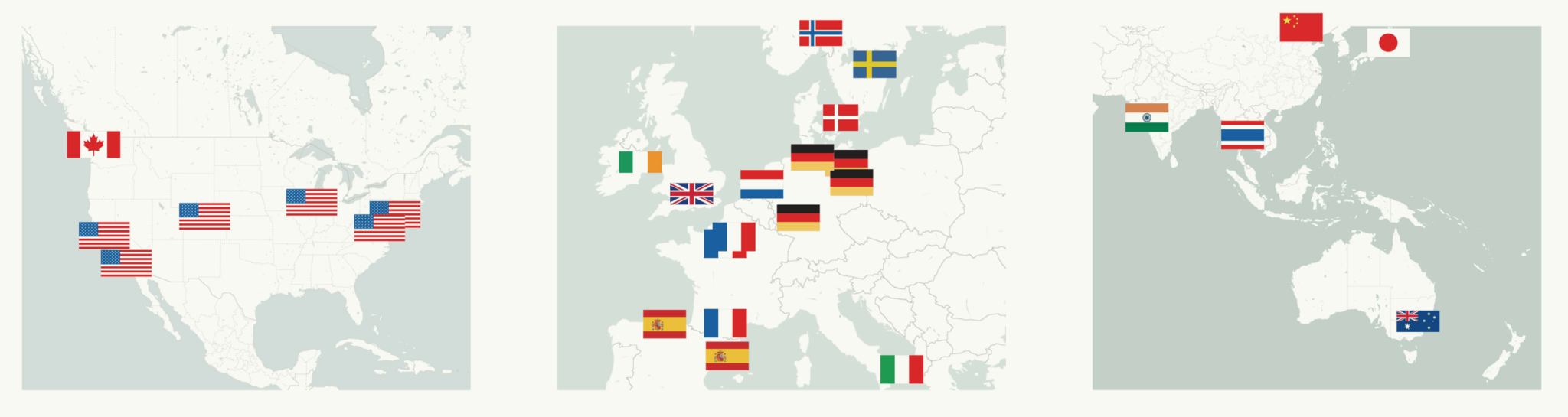
There are more than two dozen global models that comprise CMIP5. Scientists develop these models at research institutions, universities, and national laboratories around the world.
Most of the institutions that create these models share them (or their results), and the most widely used ones are made publicly available through the Coupled Model Intercomparison Project, or CMIP (pronounced see-mip). This modeling framework was first organized in 1995 by the World Climate Research Programme (WCRP), which continues to coordinate CMIPs for new generations of models. When we compare the output of these models with our own experience of the earth, we can further our understanding of our climate and how human activity affects it.
The models that are commonly referred to as climate models (and sometimes “Earth systems models”) are technically global circulation models (GCMs), because the circulation of energy, carbon, water, and other components of Earth’s systems are what drives the climate.
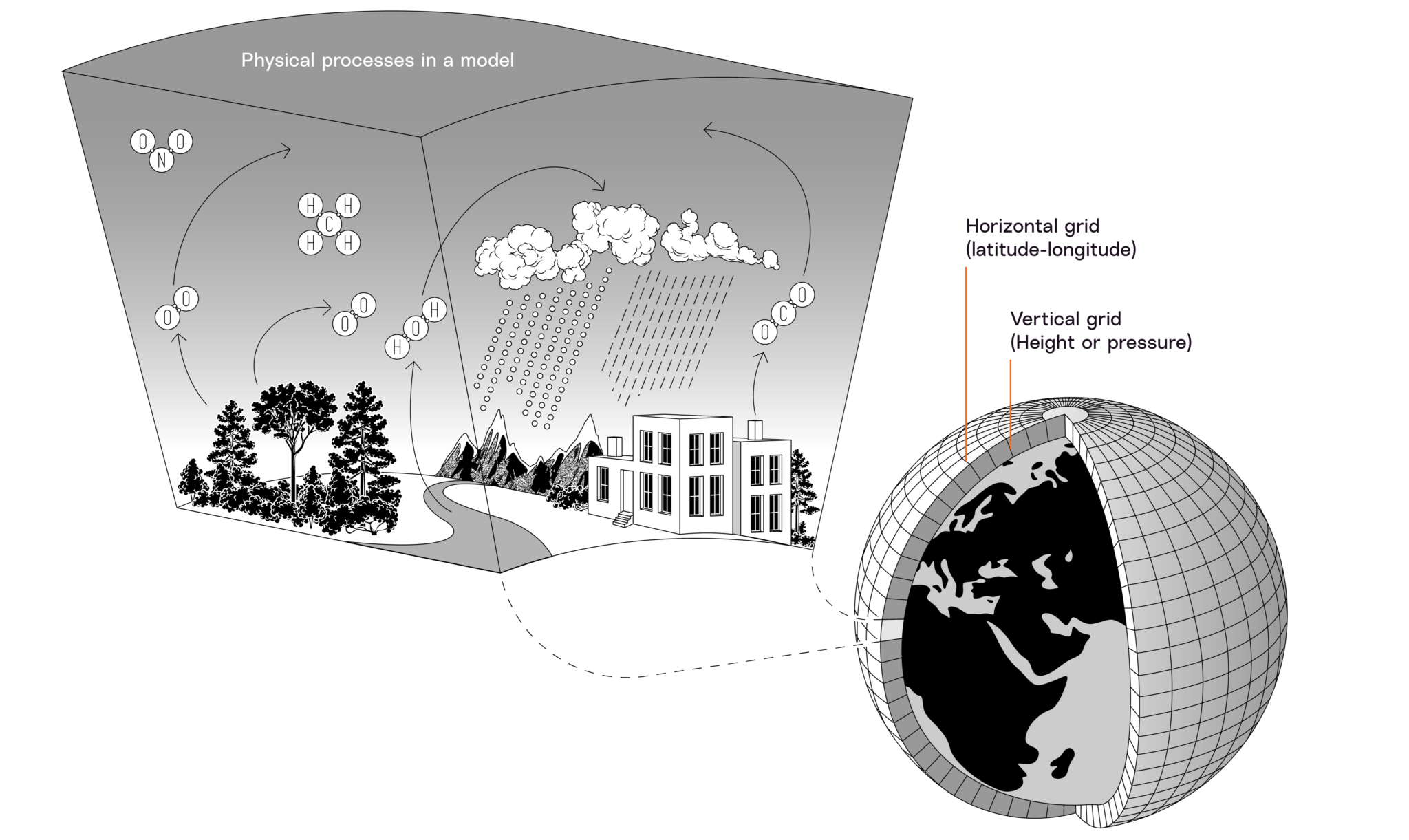
To build a model of Earth’s near-surface atmosphere, scientists divide that atmosphere into boxes called grid cells. The size of a model’s grid cells determines how detailed the results of the model will be. Most GCMs have a grid cell size of approximately 250 km on a side. RCM grid cells commonly range from 10 to 50 km to a side. Credit: Berke Yazicioglu
The models reproduce a range of physical dynamics, including ocean circulation patterns, the annual cycle of the seasons, and the flows of carbon between the land surface and the atmosphere. While weather forecasts focus on detailed local changes over hours and days, climate models project large-scale forces covering continents and spanning decades to centuries.
Every model is distinct for three reasons.
- The earth, its systems, and its weather are imperfectly and inconsistently measured. There are long, detailed, accurate data series for a few locations on Earth, but we have limited data for most of the earth even today. Each modeling team must address this incompleteness, and they may choose different ways to do so.
- Not all climate phenomena are equally well understood. Some things, like the amount of water vapor that air can hold at a given temperature, are well-known and are identical in all models. Others, including ocean currents, phenomena like El Niño and La Niña, and changes in sea ice in relation to temperature changes are subjects of ongoing research and are represented somewhat differently in different models.
- Each research group has a somewhat different area of focus. One research group might focus more effort on its modeling of glaciers, while another group might focus more on the carbon cycle of tropical forests.
The differences in these models are actually a great advantage: Since we don’t know exactly how the system works, it is better to have a range of well-informed attempts to understand it rather than only one. A few decades after scientists created the first climate models, the results confirmed this approach and have continued to do so: The average of all of the models has consistently been the most accurate in predicting how the climate would respond to changes in the atmosphere. In other words, there is no “one best model” but rather a best process for using many models.
GCMs generate numerical output for weather variables including temperature, rainfall, relative humidity, and other phenomena, for each day of a simulated year in each grid cell. GCMs themselves are not forecasts but rather simulations of outcomes given a set of conditions. Scientists can choose scenarios and run multiple models to simulate multiple years of daily conditions such as temperature and precipitation. Using modeled output, they can then calculate metrics to examine impacts like number of days in a year above 32°C (90°F) or the average temperature of the 10 hottest days of the year that would occur under the specified conditions. From the outset, CMIP models have been run for multiple emissions scenarios. While no single year will perfectly match any model’s projection, the ranges and changes in them that have occurred in the decades since the first set of models was created have closely tracked the aggregate model results of the highest emissions scenario that assumes ongoing fossil fuel use with little-to-no mitigation. The technical term for this emissions scenario is Representative Concentration Pathway 8.5, or RCP 8.5.
A GCM typically contains enough computer code to fill 18,000 pages of printed text. It takes hundreds of scientists many years to build and improve, and it requires an enormous supercomputer to run. These requirements are partly why GCMs rely on grid cells that are so large: Using smaller cells would increase modeling and computing requirements enormously.
That means that many GCM grid cells can contain very different places within them. For example, a grid cell along a coast might contain locations relatively far inland, locations right on the sea, and locations so far from shore that sailors there would be unable to spy land. GCMs only produce one value for every measure in such a cell, averaging across the various locations within it. These average results sometimes do not match the experience of any specific location that falls within the cell.
GCMs have a strong track record of portraying global and continental phenomena, but all people, animals, plants, and organisms live in specific places. Scientists use a technique called downscaling to see how different changes in the atmosphere would affect local weather. Downscaling can be approached in a few different ways, each of which carries advantages and disadvantages.
Scientists can downscale GCM results by focusing on a specific region and breaking the relevant grid cells into smaller cells. The region of focus is limited in either space or time or number of quantities simulated (e.g., daily precipitation in California); otherwise, the computational burden would be unmanageable.
- Dynamical downscaling takes the large-scale results from GCMs and uses these as boundary conditions for smaller-scale weather models. This allows scientists to better represent local topography and smaller-scale (known as mesoscale) atmospheric processes.
Dynamical downscaling improves the representation of weather dynamics particularly in topographically diverse regions. Since this type of downscaling entails running both global and regional models, it requires enormous computing power.
- Statistical downscaling breaks down larger grid cells and takes past data for the many locations inside the grid cell to create a statistical pattern consistent with the past.
For example, summer daytime temperatures tend to be cooler right on the water versus a few kilometers inland, while places at higher elevation cool off more at night—statistical downscaling accounts for this. This method requires a large amount of high quality observational data, which is not available for much of the earth. Forecasts generated using statistical downscaling techniques implicitly assume that historical relationships used to train their models will remain unchanged in the future.
Probable Futures uses both GCMs and regional climate models. RCMs are commonly used in dynamical downscaling and are much more granular than GCMs, with grid cells that range from 10 to 50 km to a side. Given the computational burden of running these detailed models, they are broken up into regions that can be run separately (e.g., South Asia, East Asia, Southeast Asia, Australasia, Africa, Europe, South America, Central America, and North America).
Days over 32°C (90°F) at 1°C of warming
-
0
-
1-7
-
8-30
-
31-90
-
91-180
-
181-365
These two maps depict the difference in data resolution between General Circulation Models (GCMs), and Regional Climate Models (RCMs). Most GCMs have a grid cell size of approximately 250km on a side. RCMs use GCM data as an input and then downscale that data using regionally-specific dynamics. This results in model output with a higher resolution. RCM grid cells commonly range from 10 to 50km on a side. Data source: CMIP5, Cordex-Core, REMO2015 and RegCM4. Processed by Woodwell Climate Research Center.
RCMs can be used effectively with GCMs. Regional models can take multiple GCM simulations of large-scale climate properties to generate finer-scale local outcomes.
While most GCMs were created using common standards through the CMIP process, RCMs were not. Research groups created RCMs and sometimes chose different GCMs to drive them, generated different outputs, and used different resolutions. The World Climate Research Programme set out to coordinate inconsistent RCM output. The resulting Coordinated Regional Climate Downscaling Experiment (CORDEX) was made public in late 2019. Since regional climate modeling is so computationally intensive, the minimum number of GCMs required for downscaling per RCM was set to three, chosen to represent a range of different climate sensitivities exhibited by the full range of GCMs.
Downscaling is an active area of research, and different methods are being developed and used by different teams of scientists. Which approach is best depends on the intended use of the model results, the availability of high-quality historical observation data from weather stations, the time horizon of interest, and budget.
Many factors have to align for GCM downscaling to paint an accurate picture of climate outcomes. Consider a group interested in one specific geographic area with a long history of accurate and complete weather observation. If researchers have access to vast amounts of computing power, they may combine dynamical and statistical downscaling of many different GCMs over many different scenarios. This strategy is particularly attractive for assessing the likelihood of extreme events.
Unfortunately, those conditions exist only in a very small number of places (e.g., California). Historical weather records are most numerous and reliable in densely populated sections of high-income countries. Data is less available in less-populated and less-developed places, and financial, scientific, and computing resources are fewer. It is thus much harder to downscale GCMs with any confidence in the results in most locations around the world. This is why RCMs were developed.
Our goal is to provide high-quality climate scenarios at different levels of warming for every place on every populated continent. With this in mind, our science partner, the Woodwell Climate Research Center (Woodwell), advised using the downscaled output from the CORDEX-CORE framework, in which two regional climate models downscale three GCMs each, and cover almost all of the populated world. The Arctic, Antarctica, and some Pacific Islands are outside of this simulated data.
- No single location has its own climate. Scientists designed climate models to project global or continental climate trends and changes. Advances including RCMs have helped to increase their resolution, but forecasting weather at the level of a specific structure or small area under a substantially different global climate not only requires myriad extra unproven assumptions, but is unlikely to be useful in strategic decisions because every structure, ecosystem, community, etc., is connected to others nearby. It is usually best to first consider model results for large areas and then zoom in to regions.
- Rising greenhouse gases impact the accuracy of models. Scientists have access to a limited amount of data to build their models, and the overwhelming majority of this data is recent. For example, consistent measures of temperature began in the mid-1800s, and satellites that collect global information first began orbiting the earth in the 1970s. As a result, climate models are particularly attuned to climatic conditions of the recent past. It is encouraging that climate models have been accurate in their predictions over the last forty years. However, the greater the concentration of greenhouse gases in the atmosphere, the further the climate gets from those foundational conditions.
In other words, the higher the warming scenario, the more uncertainty there is in the results. That is why the highest warming scenario presented on our platform is 3°C, even though we risk surpassing that level of warming without a dramatic reduction in emissions. We strongly recommend that when looking at results for 2.5°C and 3.0°C of warming, you consider the risk that the results in the models are too mild. There is inherent conservatism in climate modeling, and society faces asymmetric risks: If the models turn out to be too aggressive, it will be a nice surprise; if they turn out to be conservative, there will be much more suffering. We need to prepare for—and try to avoid—outcomes with a low probability but very high costs.
- They don’t accurately model rarer phenomena. Infrequent and complex climate events are hard to model accurately. If an event happens infrequently, scientists have limited observations to work from in creating their models. Complexity poses a related challenge. While the physical laws that govern the weather are well-understood, it is impossible to model every molecule. Indeed, even if scientists could model every molecule, there are still forms of complexity and randomness that influence outcomes (you may have heard of “the butterfly effect” wherein a small perturbation such as the flap of a butterfly’s wings sets off a chain reaction that leads to a much bigger result somewhere else in the world). That is why, for example, hurricanes and typhoons are a challenge for the modeling community, while heat is much easier to model. Scientists can draw on decades of temperature observations and have been able to use ice and sediment records to reconstruct millions of years of historical temperature data, but we only know about the hurricanes and typhoons that have been recorded, and even those are infrequent in any given location. Researchers exploring multiple approaches and methodologies for complex phenomena like storms and shifts in gulf streams will have more varied results.
- They don’t account for all biotic feedbacks. Biotic feedbacks are looped responses between terrestrial stores of carbon and the atmosphere. For example, the release of methane from organic matter in thawing permafrost and the release of CO
2
from burning forests are both caused by warming and in turn cause more warming.
It is crucial to understand that the models included in CMIP do not include large-scale atmospheric change from biotic sources. They were designed to answer questions about how human-induced atmospheric change would affect the climate. The lack of feedback loops is a critical shortcoming of the models.
- They cannot project the Hothouse Earth tipping point. The lack of these feedbacks in the models causes them to stop warming a few decades after humans stop emitting carbon. The current ensemble of models effectively assumes that we will reach a global equilibrium temperature at any given level of carbon in the atmosphere. We now know that this is unlikely even at current levels of warming and extremely unlikely at temperatures that may not sound that much warmer than now. The inability of these models to project or estimate the probability of human emissions triggering natural tipping points like runaway permafrost thaw, forest fires, and glacial melting, which could propel the planet into Hothouse Earth, is likely their biggest shortcoming.
Although biotic feedbacks are not in the historical data on which scientists have built climate models, they are a critical area of active scientific research, and one in which the Woodwell Climate Research Center is a recognized leader. Probable Futures is committed to adding biotic feedbacks to our interactive maps once the science is available, tested, and meets our own scientific standards.